However long any of us may live, there is no single moment when we actually become ‘old’. It is common to think of 60, 70, or even 80 as the start of old age — but if so, then people who reach those milestones were apparently not old a mere hour, a minute, or even a second before reaching those birthdays. The concept of a coherent beginning breaks down as soon as we begin to examine it closely.
There is no ‘first moment’ of being old, but there are definitely moments when we first realize that we are old. Acute back pain, sports injuries that refuse to heal, worrying blank spots in our memories — these jarring symptoms force us to confront the reality that our youth may be forever behind us.
Technology is another indicator. When people have trouble learning to use newer gadgets and devices (like modern remote controls or smartphones) that kids or teenagers take for granted, the age difference becomes hard to ignore. Even the way we talk about technology can divide generations:
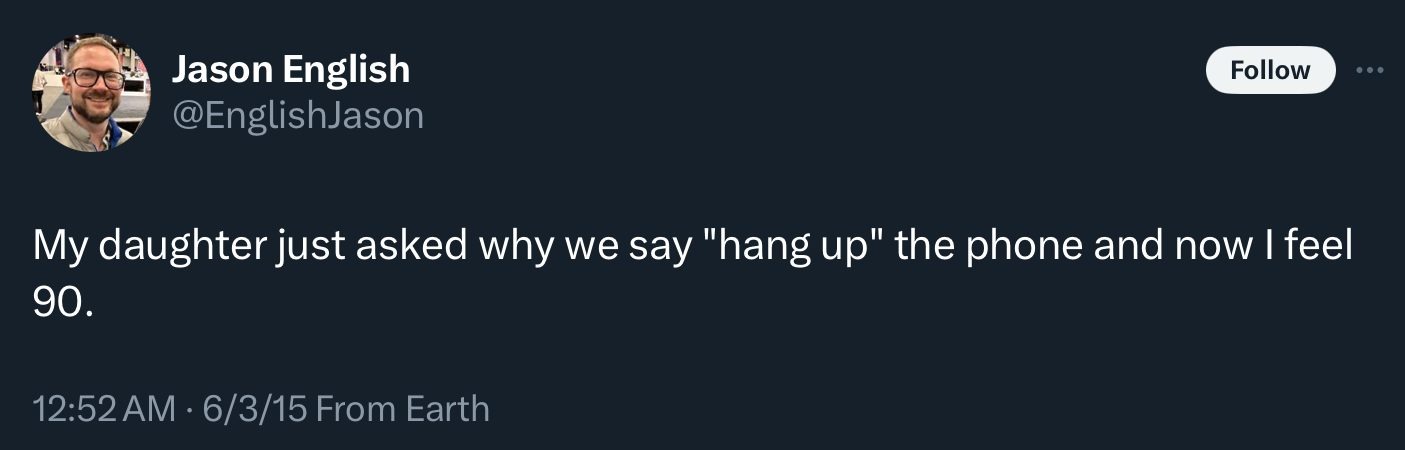
Another such generational moment is upon us. If you think you aren’t old yet, stop and ask yourself: What’s the difference between artificial intelligence, machine learning, and deep learning? Narrow AI vs Super AI? Supervised learning vs reinforcement learning?
For the younger generation, terms like these are increasingly common and soon to become second nature. The labor-intensive world we grew up with, centered around human talent, ingenuity, and muscle as the dominant engine for progress, is on its way out. To stay relevant we must learn the language of the new age, starting with the foundational concepts behind silicon-based knowledge.
Digital evolution
AI has been around for decades; even home computer users in the pre-internet era were able to play chess against their PC. AI is simply an umbrella term describing software that can perform human-like reasoning tasks. Virtual Assistants like Siri, translation programs, facial recognition tools in smartphones, and guidance systems like autopilot in airplanes and cars, fall within this broad category. Each of these may be coded entirely by humans line-by-line, or rely to a greater or lesser extent on automated data analysis, in order to function.
The AI concept is then divided along two separate axes. The first describes its level of capability. Narrow AI works to complete specific tasks, like when you use your favorite navigation app to show you the fastest way from Point A to Point B. This kind of Narrow AI program may have a perfect knowledge of city maps, but it will be useless at chess.
Artificial General Intelligence (AGI), by contrast, describes a program which can think on any topic about as well as the average human. AGI hasn’t been reached yet, and it’s unlikely to describe any product that ever will exist. The reason is that computers are already so much better than humans at certain tasks (like math), that by the time a piece of software becomes ‘as good as’ a person at everything, it will already be better than a person at many things.
That brings us to Super AI — software that exceeds human capability at a wide variety of intellectual tasks. Long a popular concept in science fiction, Super AI is now being taken much more seriously by experts in the field, many of whom think it will be a reality in less than 5 years (by which time we will probably have a better name for it).
A map of the future
AI is also categorized according to its methods. Machine learning (ML) involves the collection of large amounts of data, which is organized, categorized, and analyzed to discover useful patterns. Business software often uses this technique to process internal company data, searching for inefficiencies and suggesting improvements.
Though company data tends to be well-organized and uniformly formatted, some types of software can even sort through unstructured data. This process is called deep learning, and uses artificial neural networks to scan heterogeneous volumes of data. Large Language Models (LLMs), like ChatGPT for example, scan huge libraries of text and then learn to connect the words and phrases within them.
Machine learning can be performed in a variety of ways:
- Supervised learning involves labeled data, such as when a company analyzes its own systems.
- Unsupervised learning uses no labels for its data, forcing the algorithm to identify statistical patterns from scratch. Digital marketplaces use this technique every time they study your past purchases to make new product recommendations.
- Reinforcement learning occurs when software developers provide the algorithm with rewards for correct output and penalties for incorrect output. This method is often used in robotics; the robot’s assigned goal is to earn points, which it receives by carrying out tasks correctly and avoiding mistakes.
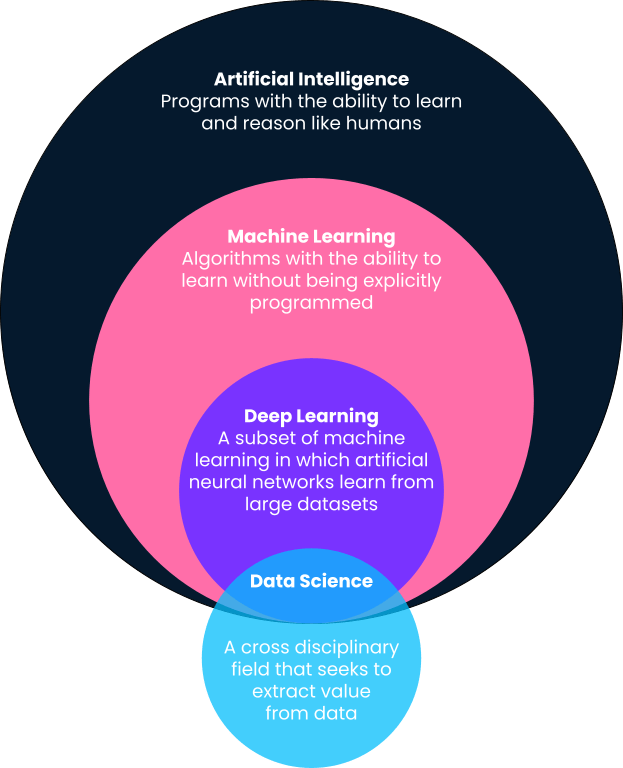
Image source: Datacamp
The list of AI-related terms and idioms is guaranteed to grow over time. We recommend getting used to the fundamental concepts now, since you’ll be hearing about them more and more in the coming years.
Plus, knowing the basics means you won’t feel old or left behind whenever AI comes up in conversation. And when age does eventually catch up with you, fear not — there will likely be a competent, charismatic, AI-driven robot to keep you company, and help take care of you, during the twilight of your years.